From Innovation to Investment: Generative AI's Role in the New Industrial Energy Era
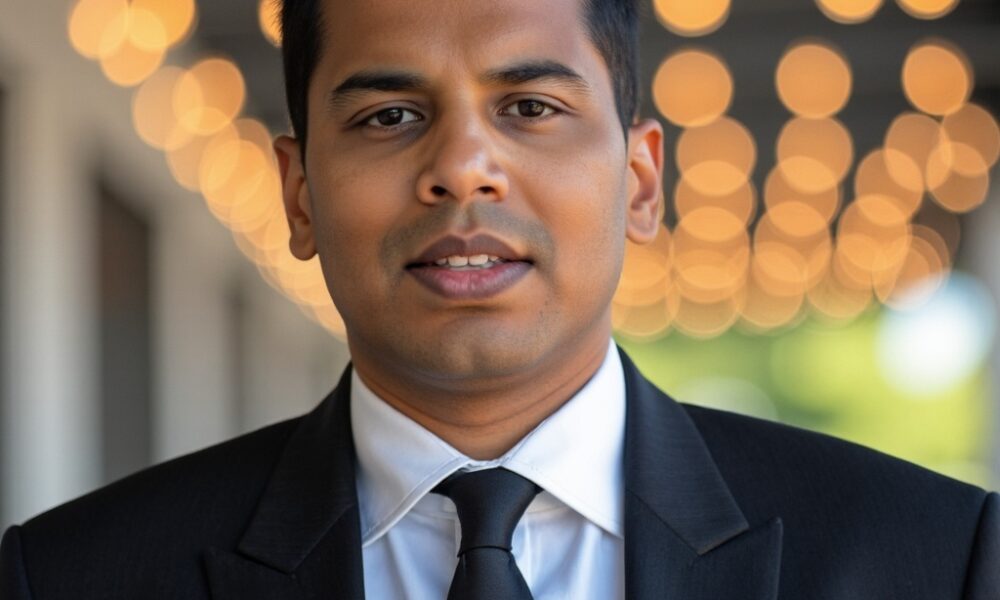
When most people hear about Generative AI, they think of viral chatbots, image creators, or office productivity tools. But there is a much bigger shift happening quietly behind the scenes. Energy, manufacturing, and industrial companies are starting to use Generative AI to rethink how they run their operations, manage their assets, and drive financial performance. It is not a theory or a far-off future. It is happening right now.
Having spent more than a decade working at the intersection of industrial markets and technology, and what I see today is a faster, deeper change than many expect. This is not about replacing jobs. It is about using AI to sharpen human judgment, improve safety, optimize capital investments, and create new sources of growth. It is helping companies move faster, waste less, and make better decisions, all of which directly tie back to margins, risk, and long-term value.
For investors, board members, and leadership teams, understanding how this technology is taking hold inside the real economy is quickly becoming a competitive edge.
Why Industrial Sectors Are Embracing Generative AI
Energy and industrial companies have always faced a difficult balancing act. They need to deliver operational excellence, manage complex assets, control costs, and now also navigate the demands of decarbonization. Layer onto that a growing talent shortage as experienced workers retire, and it is clear why companies are turning to new technologies that can multiply the capabilities of their teams.
Cloud computing has made AI at industrial scale possible. Companies can now tap into massive computational power to simulate, optimize, and predict outcomes across operations. Generative AI, in particular, brings a new dimension: the ability to create solutions, recommend actions, and design alternatives based on deep industrial data and patterns.
Unlike traditional AI models that simply detect anomalies or forecast trends, Generative AI tools can propose solutions, draft workflows, optimize maintenance plans, and even generate new engineering designs. It is an entirely different value proposition, and it is no surprise that forward-looking companies are moving to harness it.
Solutions Delivering True Impact
Several real-world applications of Generative AI are already delivering value across the energy and industrial landscape.
One of the most exciting areas is predictive maintenance. Traditional models forecast when an asset might fail, but newer AI systems go further. They suggest the best repair strategy, balancing cost, downtime, and risk. Instead of simply reacting, companies can plan smarter, allocate resources better, and extend asset lifespans.
Another promising use case is asset optimization. Refineries and plants are beginning to use AI-powered simulations to adjust operating parameters dynamically. By doing so, they are improving thermal efficiency, reducing energy consumption, and squeezing out extra margin. In many cases, these optimizations can deliver fuel savings in the range of five to ten percent, which translates into millions of dollars.
Supply chain resilience is another area seeing transformation. Generative AI models are helping companies run dynamic simulations to identify risks, evaluate suppliers, and build more robust logistics plans. In an era where disruptions are no longer rare, the ability to quickly model alternatives and adjust plans in real-time is becoming a major competitive advantage.
Finally, we are seeing a revolution in industrial design itself. AI-assisted simulation tools allow engineers to test hundreds of design variations in a fraction of the time it would take manually. Whether it is upgrading turbines, designing new battery storage systems, or optimizing refinery processes, companies that embrace these tools are accelerating innovation cycles while cutting costs.
Guidelines for Practitioners and Senior Leaders
For companies looking to apply Generative AI in industrial environments, the real work begins with data engineering long before any models are deployed. Practitioners should prioritize the curation of high-fidelity operational datasets, including time-series sensor outputs, maintenance work orders, and process simulation results. Without complete metadata, event context, and data normalization, even the most sophisticated models will fail to generalize effectively.
Model architecture choices must be driven by the business objective. For asset optimization under dynamic conditions, reinforcement learning frameworks that incorporate real-time feedback loops tend to outperform static models. On the other hand, failure mode and effects prediction often require hybrid models that blend supervised learning with first-principles physics to capture edge cases and degradation mechanisms.
For senior leadership, the focus should be on embedding AI insights into the operational technology (OT) stack, not just into information technology (IT) dashboards. Integrating predictive outputs into computerized maintenance management systems (CMMS), distributed control systems (DCS), and supply chain management platforms drives adoption at the ground level where decisions happen.
Finally, robust AI governance must be baked into deployment plans. This includes defining escalation thresholds based on risk matrices, setting drift monitoring protocols for model retraining, and building digital traceability from model inference back to operational action. Companies that treat model lifecycle management seriously will be better positioned to drive scalable, sustainable impact, rather than getting stuck in perpetual pilot mode.
The Startup Surge Driving New Innovation
Beyond the traditional players, there is a vibrant ecosystem of startups pushing the boundaries of what is possible. These startups are not just talking about AI in theory. They are building real solutions that address real operational problems. From autonomous inspections using drones and robotics to AI-driven emissions monitoring, they are moving quickly and taking fresh, practical approaches.
Venture capital and corporate investment in industrial AI startups has grown steadily over the past few years, with billions of dollars flowing into early-stage and growth-stage companies focused on energy, infrastructure, and manufacturing applications. Investors are drawn by the potential for strong returns, particularly as these startups often deliver measurable ROI within twelve to twenty-four months through cost savings, efficiency gains, and new revenue streams.
Established companies that partner with or invest in these innovators are positioning themselves not just for technological advantage, but also for financial upside. Early collaboration can lead to preferred access to new technologies, improved operational resilience, and in some cases, direct equity value creation. As Generative AI continues to move deeper into core industrial operations, the companies that act early are likely to see both operational and financial rewards.
Looking Ahead
The convergence of Generative AI, cloud computing, and deep domain expertise is setting the stage for a new industrial revolution. It will not be won by those who simply automate tasks. It will be led by those who understand how to amplify human intelligence, operational resilience, and decision speed.
For energy and industrial leaders, the message is clear. Now is the time to invest, experiment, and build capabilities around Generative AI. The risks of waiting are real. The opportunities for those who move with purpose are even greater.
The industrial future will not be built on legacy tools. It will be shaped by companies willing to imagine what comes next and ready to lead that change.
Source: From Innovation to Investment: Generative AI's Role in the New Industrial Energy Era